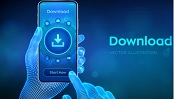

Figure 2 shows an example output by my solution with U-Net models. It can be trained end-to-end from few images and outperform the prior best method on the ISBI cell tracking challenge 2015. U-Net is one of the most successful and popular convolutional neural network architecture for medical image segmentation. My model is based on a variant of fully convolutional neural network, U-Net, which is developed by.

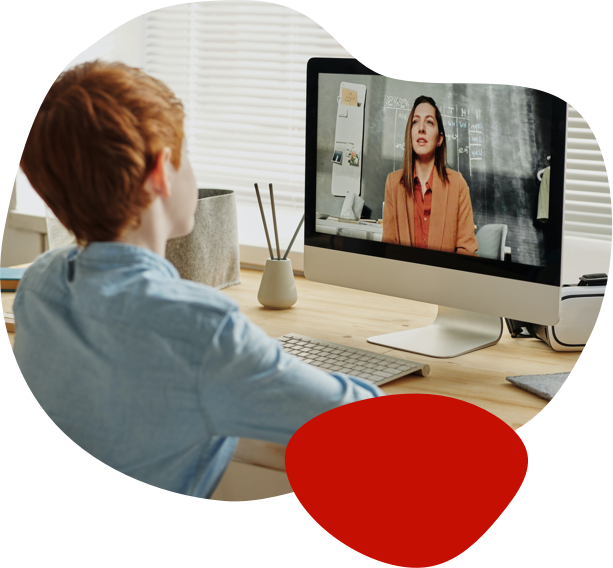
I solved the problem as a semantic segmentation task in computer vision. I individually investigated and evaluated the similar approach on Spacenet Challenge dataset (my first submission with the approach is on May 13, a little earlier than their publication). , published on May 17, also investigates the use of OpenStreetMap for semantic labeling of satellite image. My best individual model simply uses OpenStreetMap layers and multispectral layers as the input of the deep neural network simultaneously (as described in Figure1).įigure 1: Best individual model with using OpenStreetMap and Pan-sharpened Multispectral data. In addition, I found the use of OpenStreetMap data is effective for predicting the building footprint. My final submission is the averaging ensemble from individually trained three U-Net models. I applied a modified U-Net model, one of deep neural network model for image segmentation. The polygon of building footprint proposed by the algorithm is considered as a true positive if its IOU (Intersection over Union, Jaccard index) score is higher than 0.5. The algorithm is evaluated based on F-score. SpaceNet Challenge Round2 asks its participants to submit an algorithm that inputs satellite images (of Las Vegas, Paris, Shanghai and Khartoum) and outputs polygons of building footprints. It consists of an online repository of freely available satellite imagery, co-registered map layers to train algorithms, and public challenges that aim to accelerate innovation in machine leanring.

SpaceNet is a collaboration between DigitalGlobe (a commercial vendor of space imagery and geospatial content), CosmiQ Works (a division of In-Q-Tel Lab) and NVIDIA (the world leading company in visual computing technologies). For training a deep neural network model, the computational time on p2.xlarge (Tesla K80) is two times longer than my personal graphic card (GeForce GTX 1080).Adding OpenStreetMap layers into the input of U-Net model significantly improves F-score.Furthermore, we think that solving this challenge is an important stepping stone to unleashing the power of advanced computer vision algorithms applied to a variety of remote sensing data applications in both the public and private sector. We believe that advancing automated feature extraction techniques will serve important downstream uses of map data including humanitarian and disaster response, as observed by the need to map road networks during the response to recent flooding in Bangladesh and Hurricane Maria in Puerto Rico.
#Topcoder spacenet manual
Today, map features such as roads, building footprints, and points of interest are primarily created through manual techniques. CosmiQ Works, Radiant Solutions and NVIDIA have partnered to release the SpaceNet data set to the public to enable developers and data scientists to work with this data. One area for innovation is the application of computer vision and deep learning to extract information from satellite imagery at scale. The commercialization of the geospatial industry has led to an explosive amount of data being collected to characterize our changing planet.
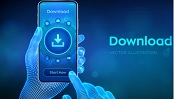